This is completed downloadable of Applied Multivariate Statistical Analysis 6th Edition Johnson Solutions Manual
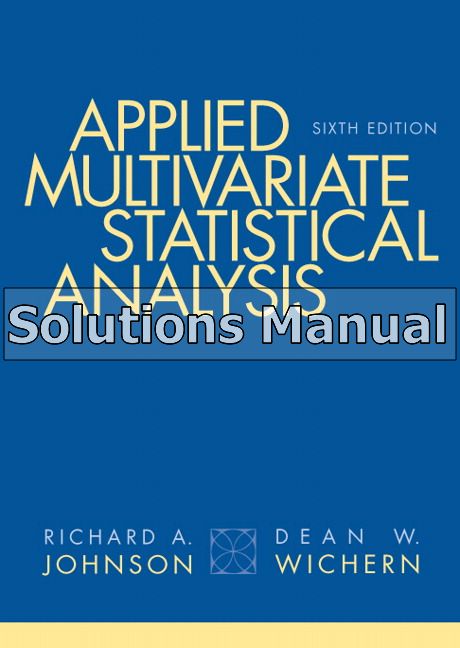
Product Details:
This classroom-tested text offers a readable introduction to the statistical analysis of multivariate observations. Its primary goal is to impart the necessary knowledge to make proper interpretations and select appropriate techniques for analyzing multivariate data.
It is suitable for courses in Multivariate Statistics, Marketing Research, Statistics in Education and postgraduate-level courses in Experimental Design and Statistics.
Table of Content:
I. GETTING STARTED
- 1. Aspects of Multivariate Analysis
- Applications of Multivariate Techniques. The Organization of Data. Data Displays and Pictorial Representations. Distance. Final Comments.
- 2. Matrix Algebra and Random Vectors
- Some Basics of Matrix and Vector Algebra. Positive Definite Matrices. A Square-Root Matrix. Random Vectors and Matrices. Mean Vectors and Covariance Matrices. Matrix Inequalities and Maximization. Supplement 2A Vectors and Matrices: Basic Concepts.
- 3. Sample Geometry and Random Sampling
- The Geometry of the Sample. Random Samples and the Expected Values of the Sample Mean and Covariance Matrix. Generalized Variance. Sample Mean, Covariance, and Correlation as Matrix Operations. Sample Values of Linear Combinations of Variables.
- 4. The Multivariate Normal Distribution
- The Multivariate Normal Density and Its Properties. Sampling from a Multivariate Normal Distribution and Maximum Likelihood Estimation. The Sampling Distribution of `X and S. Large-Sample Behavior of `X and S. Assessing the Assumption of Normality. Detecting Outliners and Data Cleaning. Transformations to Near Normality.
II. INFERENCES ABOUT MULTIVARIATE MEANS AND LINEAR MODELS
- 5. Inferences About a Mean Vector
- The Plausibility of …m0 as a Value for a Normal Population Mean. Hotelling’s T2 and Likelihood Ratio Tests. Confidence Regions and Simultaneous Comparisons of Component Means. Large Sample Inferences about a Population Mean Vector. Multivariate Quality Control Charts. Inferences about Mean Vectors When Some Observations Are Missing. Difficulties Due To Time Dependence in Multivariate Observations. Supplement 5A Simultaneous Confidence Intervals and Ellipses as Shadows of the p-Dimensional Ellipsoids.
- 6. Comparisons of Several Multivariate Means
- Paired Comparisons and a Repeated Measures Design. Comparing Mean Vectors from Two Populations. Comparison of Several Multivariate Population Means (One-Way MANOVA). Simultaneous Confidence Intervals for Treatment Effects. Two-Way Multivariate Analysis of Variance. Profile Analysis. Repealed Measures, Designs, and Growth Curves. Perspectives and a Strategy for Analyzing Multivariate Models.
- 7. Multivariate Linear Regression Models
- The Classical Linear Regression Model. Least Squares Estimation. Inferences About the Regression Model. Inferences from the Estimated Regression Function. Model Checking and Other Aspects of Regression. Multivariate Multiple Regression. The Concept of Linear Regression. Comparing the Two Formulations of the Regression Model. Multiple Regression Models with Time Dependant Errors. Supplement 7A The Distribution of the Likelihood Ratio for the Multivariate Regression Model.
III. ANALYSIS OF A COVARIANCE STRUCTURE
- 8. Principal Components
- Population Principal Components. Summarizing Sample Variation by Principal Components. Graphing the Principal Components. Large-Sample Inferences. Monitoring Quality with Principal Components. Supplement 8A The Geometry of the Sample Principal Component Approximation.
- 9. Factor Analysis and Inference for Structured Covariance Matrices
- The Orthogonal Factor Model. Methods of Estimation. Factor Rotation. Factor Scores. Perspectives and a Strategy for Factor Analysis. Structural Equation Models. Supplement 9A Some Computational Details for Maximum Likelihood Estimation.
- 10. Canonical Correlation Analysis
- Canonical Variates and Canonical Correlations. Interpreting the Population Canonical Variables. The Sample Canonical Variates and Sample Canonical Correlations. Additional Sample Descriptive Measures. Large Sample Inferences.
IV. CLASSIFICATION AND GROUPING TECHNIQUES
- 11. Discrimination and Classification
- Separation and Classification for Two Populations. Classifications with Two Multivariate Normal Populations. Evaluating Classification Functions. Fisher’s Discriminant Function…ñSeparation of Populations. Classification with Several Populations. Fisher’s Method for Discriminating among Several Populations. Final Comments.
- 12. Clustering, Distance Methods and Ordination
- Similarity Measures. Hierarchical Clustering Methods. Nonhierarchical Clustering Methods. Multidimensional Scaling. Correspondence Analysis. Biplots for Viewing Sample Units and Variables. Procustes Analysis: A Method for Comparing Configurations.
Appendix
- Standard Normal Probabilities. Student’s t-Distribution Percentage Points. …c2 Distribution Percentage Points. F-Distribution Percentage Points. F-Distribution Percentage Points (…a = .10). F-Distribution Percentage Points (…a = .05). F-Distribution Percentage Points (…a = .01).
Data Index
Subject Index
People Also Search:
applied multivariate statistical analysis johnson
applied multivariate statistical analysis 6th edition johnson
applied multivariate statistical analysis 6th edition download scribd
applied multivariate statistical analysis 6th edition solution manual download pdf
applied multivariate statistical analysis 6th edition
Instant download after Payment is complete